Distributions with Two Random Variables#
Plotting the empirical PDF of \( \mathbf{z} \) (where \( \mathbf{z} = \mathbf{x} + \mathbf{y} \)) and compare it with the theoretical PDF derived from the convolution of the PDFs of \( \mathbf{x} \) and \( \mathbf{y} \).
Generate the Random Variables#
We’ll start by generating two independent random variables \( \mathbf{x} \) and \( \mathbf{y} \), each following a Gaussian distribution with zero mean and unit variance. Then we’ll compute \( \mathbf{z} = \mathbf{x} + \mathbf{y} \).
Plot the Empirical PDF of \( \mathbf{z} \)#
We’ll create a histogram of the data for \( \mathbf{z} \) and normalize it to represent the empirical PDF.
Calculate the Theoretical PDF of \( \mathbf{z} \)#
Since \( \mathbf{z} \) is the sum of two independent Gaussian random variables, its PDF can be calculated as the convolution of the individual PDFs of \( \mathbf{x} \) and \( \mathbf{y} \). Given that both \( \mathbf{x} \) and \( \mathbf{y} \) are Gaussian, the theoretical PDF of \( \mathbf{z} \) will also be Gaussian with mean \( 0 \) and variance \( 2 \) (since the variance of the sum is the sum of the variances).
import numpy as np
import matplotlib.pyplot as plt
from scipy.stats import norm
from scipy.signal import convolve
def generate_joint_gaussian_pdf(num_samples):
# Generate independent Gaussian random variables
x = np.random.normal(0, 1, num_samples)
y = np.random.normal(0, 1, num_samples)
z = x + y
# Define the grid for the histogram
x_bins = np.linspace(-5, 5, 50)
y_bins = np.linspace(-5, 5, 50)
X, Y = np.meshgrid(x_bins, y_bins)
# Compute the 2D histogram for the joint PDF
hist, x_edges, y_edges = np.histogram2d(x, y, bins=(x_bins, y_bins), density=True)
# Meshgrid for the bins
X, Y = np.meshgrid(x_edges[:-1], y_edges[:-1])
# Plot the 3D surface of the joint PDF
fig = plt.figure(figsize=(10, 8))
ax = fig.add_subplot(111, projection='3d')
ax.plot_surface(X, Y, hist, cmap='viridis')
# Label the axes
ax.set_xlabel('x')
ax.set_ylabel('y')
ax.set_zlabel('p_X_Y(x, y)')
ax.set_title('3D Joint PDF of z = x + y')
plt.show()
# Step 2: Plot the empirical PDF of z
plt.figure(figsize=(10, 6))
plt.hist(z, bins=50, density=True, alpha=0.6, color='blue', label='Empirical PDF of z')
# Step 3: Compute and plot the convolution of PDFs of x and y
z_range = np.linspace(-10, 10, 1000)
pdf_x = norm.pdf(z_range, loc=0, scale=1)
pdf_y = norm.pdf(z_range, loc=0, scale=1)
# Convolution of pdf_x and pdf_y
pdf_z_convolution = convolve(pdf_x, pdf_y, mode='same') * (z_range[1] - z_range[0])
plt.plot(z_range, pdf_z_convolution, 'r-', linewidth=2, label=r'Convolution of PDFs of x and y')
# Add labels and legend
plt.xlabel('z')
plt.ylabel('PDF')
plt.title('Empirical PDF of z and Theoretical Convolution of PDFs of x and y')
plt.legend()
plt.grid(True)
plt.show()
# Example usage
generate_joint_gaussian_pdf(10000)
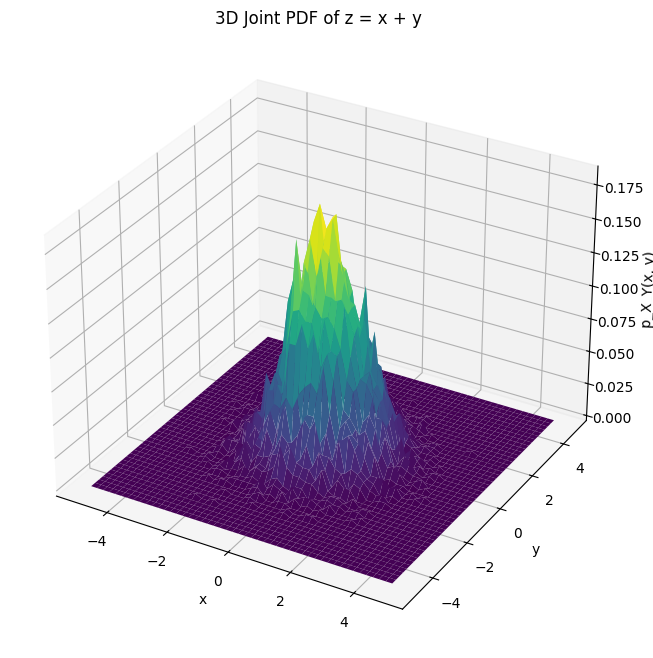
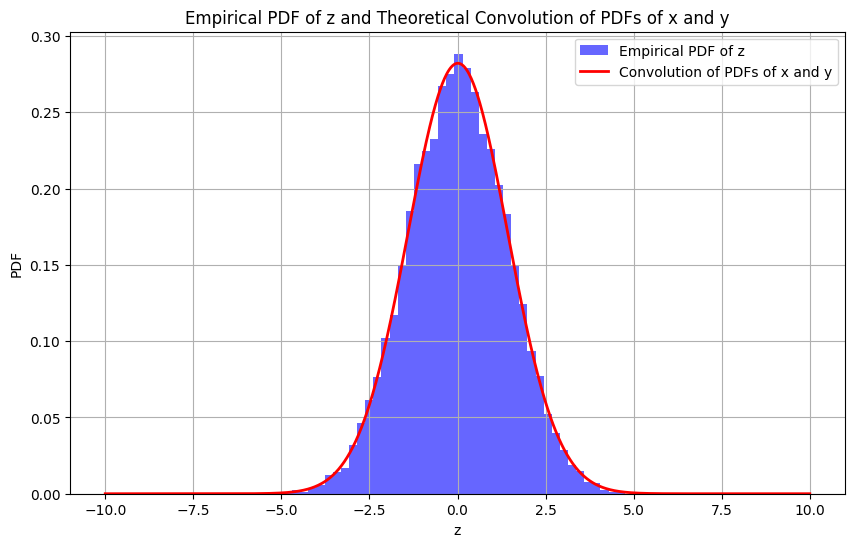
Schonhoff’s Method#
This code generates a 2-dimensional (2D) joint Gaussian random variable (RV) and then computes and displays its empirical joint probability density function (PDF).
The random variables (RVs) generated are statistically independent, have zero mean, and unit variance.
The empirical PDF is obtained using a histogram-based method.
import numpy as np
import matplotlib.pyplot as plt
def generate_joint_gaussian_pdf(num):
"""
Generate the empirical joint Gaussian PDF.
Parameters:
num (int): Number of random variables (RVs) to generate.
Returns:
numpy.ndarray: The empirical joint Gaussian PDF.
"""
# Initialize arrays for storing the generated random variables
x = np.zeros(num)
y = np.zeros(num)
# Generate the random variables using the Box-Muller transform
for i in range(num):
ui = np.random.rand()
uq = np.random.rand()
x[i] = np.sqrt(-2 * np.log(ui)) * np.cos(2 * np.pi * uq)
y[i] = np.sqrt(-2 * np.log(ui)) * np.sin(2 * np.pi * uq)
# Define the bins for the histogram
x_bins = np.arange(-5, 5.5, 0.5)
y_bins = np.arange(-5, 5.5, 0.5)
# Compute the histograms
nx, _ = np.histogram(x, bins=x_bins)
ny, _ = np.histogram(y, bins=y_bins)
# Compute the joint histogram (empirical joint PDF)
joint_pdf = np.outer(nx, ny)
# Normalize the joint histogram to approximate the joint PDF
joint_pdf = joint_pdf * 4 / 1e8
# Plotting the joint PDF
X, Y = np.meshgrid(x_bins[:-1], y_bins[:-1])
fig = plt.figure()
ax = fig.add_subplot(111, projection='3d')
ax.plot_surface(X, Y, joint_pdf, cmap='viridis')
ax.set_xlabel('x')
ax.set_ylabel('y')
ax.set_zlabel('p_X_Y(x,y)')
plt.show()
return joint_pdf # Ensure that the output is not printed automatically by assigning to a variable
# Example usage
# Call the function but do not display the result
_ = generate_joint_gaussian_pdf(1000)
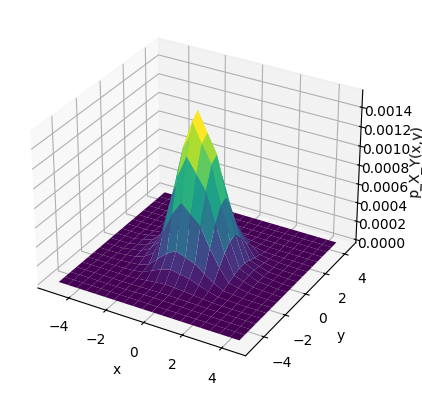