Rician Random Variable#
A Rician random variable is closely related to the Rayleigh random variable (in fact, the Rayleigh distribution is a special case of the Rician distribution).
The probability density function (PDF) of a Rician random variable is given (for any \(a > 0\) and any \(\sigma > 0\)) by:
In this expression, the function \(I_0(x)\) is the modified Bessel function of the first kind of order zero, which is defined by:
In the context of the Rician distribution, the parameters \(a\) and \(\sigma\) have the following names and interpretations:
Non-Centrality Parameter \(a\)
This is the signal amplitude parameter or the non-centrality parameter.
It represents the deterministic component of the signal or the mean of the underlying complex Gaussian random variable.
Physically, in communication systems, \(a\) often represents the strength of the line-of-sight (LOS) component of a signal.
Scale Parameter \(\sigma\)
This is the scale parameter or the standard deviation of the underlying Gaussian noise components.
It represents the spread or variance of the random (non-LOS) components of the signal, which arise from scattering or noise.
Together, \(a\) and \(\sigma\) characterize the Rician fading environment, where \(a = 0\) corresponds to the special case of the Rayleigh distribution (pure non-LOS signal).
Like the Gaussian random variable, the cumulative distribution function (CDF) of a Rician random variable cannot be written in closed form.
Similar to the Q-function used to describe the Gaussian CDF, there is another function known as Marcum’s Q-function, which describes the CDF of a Rician random variable. It is defined by:
The CDF of the Rician random variable is then given by:
Simulation of PDF#
import numpy as np
import matplotlib.pyplot as plt
from scipy.stats import rice, rayleigh
from scipy.special import i0
# Define the theoretical Rician PDF
def rician_pdf(x, a, sigma):
return (x / sigma**2) * np.exp(-(x**2 + a**2) / (2 * sigma**2)) * i0(a * x / sigma**2)
# Simulation parameters
num_samples = 100000 # Number of samples for empirical PDF
x_values = np.linspace(0, 10, 1000) # X-axis for PDF
a_values = [0.5, 2, 4] # Different a values
sigma = 1 # Fixed sigma for simplicity
# Plot PDFs for different a values and Rayleigh
plt.figure(figsize=(12, 8))
for a in a_values:
# Simulate Rician random variable
samples = rice.rvs(a / sigma, scale=sigma, size=num_samples)
# Compute empirical PDF
hist, bin_edges = np.histogram(samples, bins=50, density=True)
bin_centers = (bin_edges[:-1] + bin_edges[1:]) / 2
# Compute theoretical PDF
theoretical_pdf = rician_pdf(x_values, a, sigma)
# Plot empirical PDF
plt.plot(bin_centers, hist, label=f"Empirical PDF (a={a})", linestyle="dashed")
# Plot theoretical PDF
plt.plot(x_values, theoretical_pdf, label=f"Theoretical PDF (a={a})")
# Add the Rayleigh distribution (special case of Rician when a=0)
rayleigh_pdf = rayleigh.pdf(x_values, scale=sigma)
plt.plot(x_values, rayleigh_pdf, label="Rayleigh PDF", linestyle="dashdot", color="black")
# Customize plot
plt.title("Empirical vs Theoretical PDF of Rician Distribution with Rayleigh PDF", fontsize=16)
plt.xlabel("x", fontsize=14)
plt.ylabel("PDF", fontsize=14)
plt.legend(fontsize=12)
plt.grid(True)
plt.show()
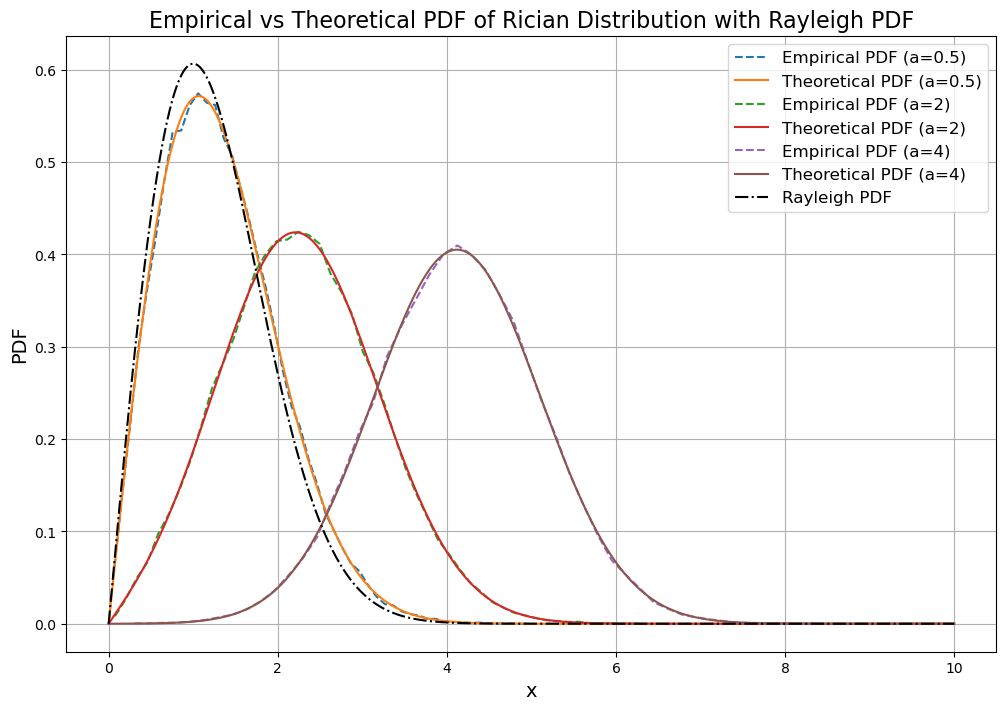
Simulation of CDF#
from marcumq import marcumq
import numpy as np
import matplotlib.pyplot as plt
from scipy.stats import rice
# Define the theoretical CDF of the Rician distribution
def rician_cdf(x, a, sigma):
a_temp = a / sigma
b_temp = x / sigma
return 1 - marcumq(1, a_temp, b_temp)
# Simulation parameters
a_values = [0.5, 2, 4] # Different a values for CDF
num_samples = 100000 # Number of samples for empirical CDF
x_values = np.linspace(0, 10, 1000) # X-axis for CDF
sigma = 1 # Fixed sigma
# Plot CDFs for different a
plt.figure(figsize=(12, 8))
for a in a_values:
# Simulate Rician random variable
samples = rice.rvs(a / sigma, scale=sigma, size=num_samples)
# Compute empirical CDF using a histogram (bar representation)
hist, bin_edges = np.histogram(samples, bins=50, density=True)
cdf = np.cumsum(hist * np.diff(bin_edges)) # Normalize by bin width for proper CDF
# Compute theoretical CDF
theoretical_cdf = [rician_cdf(x, a, sigma) for x in x_values]
# Plot empirical CDF as a bar plot
bin_centers = (bin_edges[:-1] + bin_edges[1:]) / 2
plt.bar(bin_centers, cdf, width=np.diff(bin_edges), alpha=0.4, label=f"Empirical CDF (a={a})")
# Plot theoretical CDF
plt.plot(x_values, theoretical_cdf, label=f"Theoretical CDF (a={a})")
# Customize plot
plt.title("Empirical vs Theoretical CDF of Rician Distribution", fontsize=16)
plt.xlabel("x", fontsize=14)
plt.ylabel("CDF", fontsize=14)
plt.legend(fontsize=12)
plt.grid(True)
plt.show()
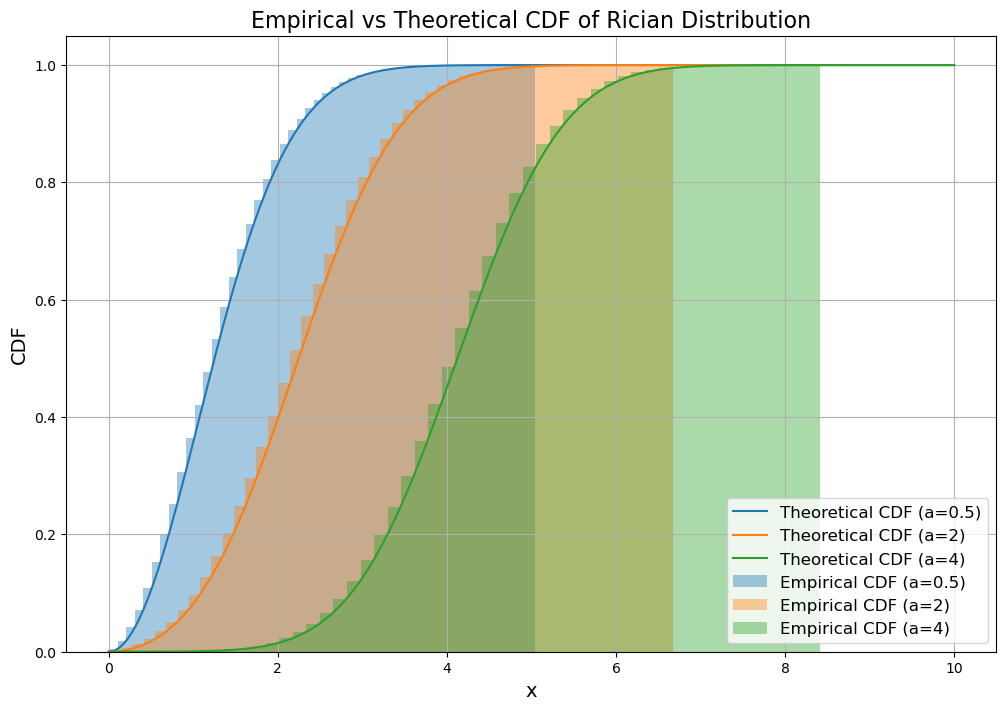
References#
P. Z. Peebles Jr., “Probability, Random Variables, and Random Signal Principles,” 4rd Edition, McGraw-Hill Book Co., 2001.