Example: Band-Limited White Gaussian Noise Process#
In this example, assume that the autocorrelation of a zero-mean, real Gaussian process \( \mathbf{x}(t) \) is given by
Let \( \mathbf{v}(t) = \mathbf{x}^2(t) \).
The autocorrelation and power spectral density of the process \( \mathbf{v}(t) = \mathbf{x}^2(t) \) are determined in this example.
The autocorrelation function of \( \mathbf{v}(t) \) is given by
The corresponding power spectral density is
where
and
Process \(\mathbf{x}(t)\)#
We will start by interpreting the provided autocorrelation function and the power spectral density.
Autocorrelation Function#
The autocorrelation function \( R_{\mathbf{x}}(\tau) \) is given by:
This function is commonly known as the sinc function, which is a characteristic of a band-limited process.
Power Spectral Density (PSD)#
The power spectral density \( S_{\mathbf{x}}(f) \) is provided as:
This PSD indicates that the process \( x(t) \) is band-limited to \( B/2 \) Hz, and it has a flat spectrum within this band.
Interpreting the Process \( \mathbf{x}(t) \)#
Given that \( \mathbf{x}(t) \) is a zero-mean, real Gaussian process with the specified autocorrelation and PSD, we can conclude that \( \mathbf{x}(t) \) is a band-limited white Gaussian noise process. The sinc autocorrelation function suggests a band-limited process, and the flat PSD indicates that within the band \( |f| < B/2 \), the power is constant.
import numpy as np
from scipy.signal import butter, lfilter
from scipy.signal.windows import hamming
import matplotlib.pyplot as plt
import warnings
warnings.filterwarnings("ignore")
# Parameters
B = 2 # Bandwidth in Hz
N0 = 1 # Power spectral density level
Fs = 10 # Sampling frequency in Hz
T = 20 # Duration of the signal in seconds
N = int(T * Fs) # Number of samples
# Time vector
t = np.arange(N) / Fs
# Generate white Gaussian noise
x = np.sqrt(N0 * Fs) * np.random.randn(N)
# Design a low-pass filter to band-limit the noise
f_cutoff = B / 2 # Cutoff frequency
b, a = butter(6, f_cutoff / (Fs / 2)) # 6th order Butterworth filter
# Apply the filter to the noise
x_bandlimited = lfilter(b, a, x)
# Compute the empirical PSD using FFT
X = np.fft.fft(x_bandlimited, n=N)
PSD_empirical = (1 / (Fs * N)) * np.abs(X) ** 2
PSD_empirical = np.fft.fftshift(PSD_empirical) # Shift zero frequency component to the center
f = np.fft.fftshift(np.fft.fftfreq(N, d=1/Fs)) # Frequency vector for both positive and negative frequencies
# Theoretical PSD
PSD_theoretical = 2 * N0 * (np.abs(f) <= B / 2)
# Plotting
plt.figure()
plt.plot(f, PSD_empirical, 'b', linewidth=1.5, label='Empirical PSD')
plt.plot(f, PSD_theoretical, 'r--', linewidth=1.5, label='Theoretical PSD')
plt.xlabel('Frequency (Hz)')
plt.ylabel('Power/Frequency (dB/Hz)')
plt.title('Empirical vs Theoretical PSD')
plt.legend()
plt.grid(True)
plt.show()
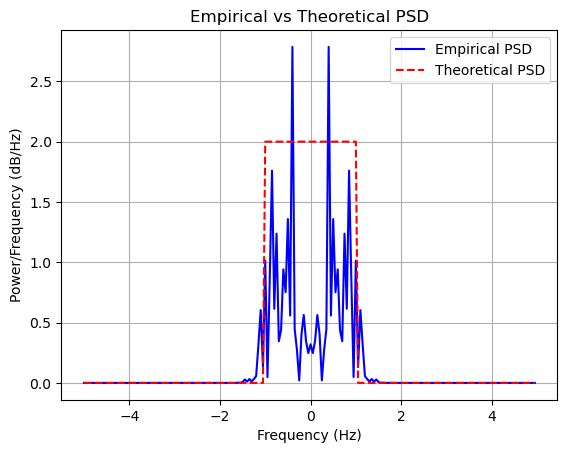
import numpy as np
from scipy.signal import butter, lfilter
from scipy.signal.windows import hamming
import matplotlib.pyplot as plt
import warnings
warnings.filterwarnings("ignore")
# Parameters
B = 2 # Bandwidth in Hz
N0 = 1 # Power spectral density level
Fs = 10 # Sampling frequency in Hz
T = 20 # Duration of the signal in seconds
N = int(T * Fs) # Number of samples
# Time vector
t = np.arange(N) / Fs
# Generate white Gaussian noise
x = np.sqrt(N0 * Fs) * np.random.randn(N)
# Design a low-pass filter to band-limit the noise
f_cutoff = B / 2 # Cutoff frequency
b, a = butter(6, f_cutoff / (Fs / 2)) # 6th order Butterworth filter
# Apply the filter to the noise
x_bandlimited = lfilter(b, a, x)
# Compute the empirical PSD using FFT
# New method to compute the PSD based on Schonhoff's method, Fig. 2.8
X = np.fft.fft(x_bandlimited, n=N)
PSD_empirical = (1 / (Fs * N)) * np.abs(X) ** 2
PSD_empirical = np.fft.fftshift(PSD_empirical) # Shift zero frequency component to the center
f = np.fft.fftshift(np.fft.fftfreq(N, d=1/Fs)) # Frequency vector for both positive and negative frequencies
# Theoretical PSD
PSD_theoretical = 2 * N0 * (np.abs(f) <= B / 2)
# Inverse Fourier Transform to get the autocorrelation function
autocorr_empirical = np.fft.ifftshift(np.fft.ifft(np.fft.ifftshift(PSD_empirical)))
# Plotting the Empirical PSD vs Theoretical PSD
plt.figure()
plt.plot(f, PSD_empirical, 'b', linewidth=1.5, label='Empirical PSD')
plt.plot(f, PSD_theoretical, 'r--', linewidth=1.5, label='Theoretical PSD')
plt.xlabel('Frequency (Hz)')
plt.ylabel('Power/Frequency (dB/Hz)')
plt.title('Empirical vs Theoretical PSD')
plt.legend()
plt.grid(True)
plt.show()
# Plotting the Autocorrelation Function
plt.figure()
plt.plot(t - T/2, np.real(autocorr_empirical), linewidth=1.5, label='Autocorrelation (Empirical)')
plt.xlabel('Time Lag (s)')
plt.ylabel('Autocorrelation')
plt.title('Autocorrelation Function from Empirical PSD')
plt.legend()
plt.grid(True)
plt.show()
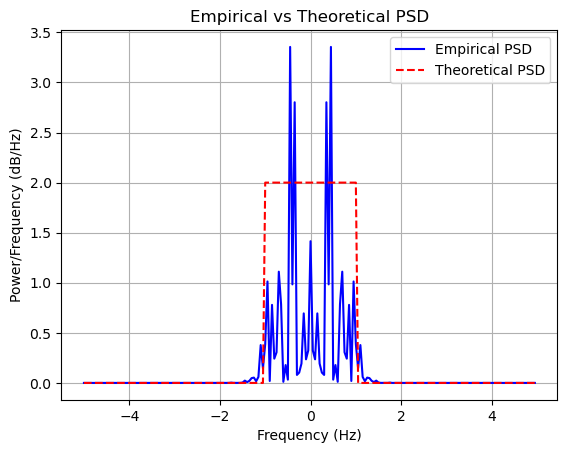
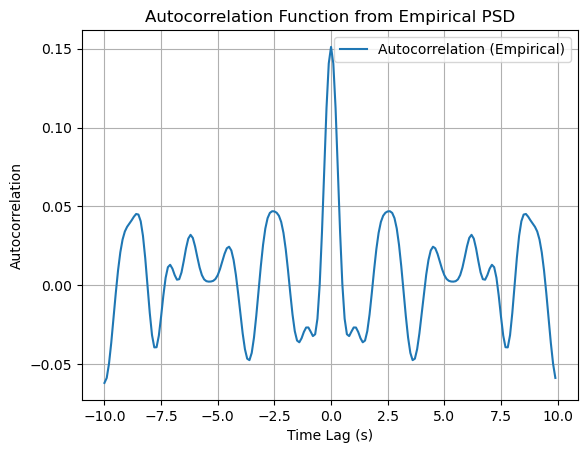
Process \(\mathbf{v}(t)\)#
To simulate the process \(\mathbf{v}(t) = \mathbf{x}^2(t)\), compute its empirical PSD, and plot the theoretical PSD, we will proceed with the following steps in Python:
Generate \(\mathbf{x}(t)\) as a band-limited Gaussian noise process.
Generate \(\mathbf{v}(t)\) as the square of \(\mathbf{x}(t)\).
Compute the empirical PSD of \(\mathbf{v}(t)\).
Calculate the theoretical PSD of \(\mathbf{v}(t)\) and plot it for comparison.
import numpy as np
from scipy.signal import butter, lfilter
import matplotlib.pyplot as plt
# Parameters
B = 2 # Bandwidth in Hz
N0 = 1 # Power spectral density level
Fs = 10 # Sampling frequency in Hz
T = 20 # Duration of the signal in seconds
N = int(T * Fs) # Number of samples
# Time vector
t = np.arange(N) / Fs
# Generate white Gaussian noise
x = np.sqrt(N0 * Fs) * np.random.randn(N)
# Design a low-pass filter to band-limit the noise
f_cutoff = B / 2 # Cutoff frequency
b, a = butter(6, f_cutoff / (Fs / 2)) # 6th order Butterworth filter
# Apply the filter to the noise
x_bandlimited = lfilter(b, a, x)
# Generate v(t) = x^2(t)
v = x_bandlimited**2
# Compute the empirical PSD of v(t) using FFT
V = np.fft.fft(v, n=N)
PSD_empirical_v = (1 / (Fs * N)) * np.abs(V) ** 2
PSD_empirical_v = np.fft.fftshift(PSD_empirical_v) # Shift zero frequency component to the center
f = np.fft.fftshift(np.fft.fftfreq(N, d=1/Fs)) # Frequency vector for both positive and negative frequencies
# Theoretical PSD of v(t)
# C(f) calculation
def theoretical_psd_x(f):
return 2 * N0 * (np.abs(f) < B / 2)
def C(f):
f_range = np.linspace(-Fs/2, Fs/2, N)
S_x_f = theoretical_psd_x(f_range)
S_x_f_shifted = theoretical_psd_x(f[:, None] - f_range)
return np.trapz(S_x_f * S_x_f_shifted, f_range, axis=-1)
S_v_f = (2 * N0 * B) ** 2 * (f == 0) + 2 * C(f)
# Plotting
plt.figure()
plt.plot(f, PSD_empirical_v, 'b', linewidth=1.5, label='Empirical PSD of v(t)')
plt.plot(f, S_v_f, 'r--', linewidth=1.5, label='Theoretical PSD of v(t)')
plt.xlabel('Frequency (Hz)')
plt.ylabel('Power/Frequency (dB/Hz)')
plt.title('Empirical vs Theoretical PSD of v(t)')
plt.legend()
plt.grid(True)
plt.show()
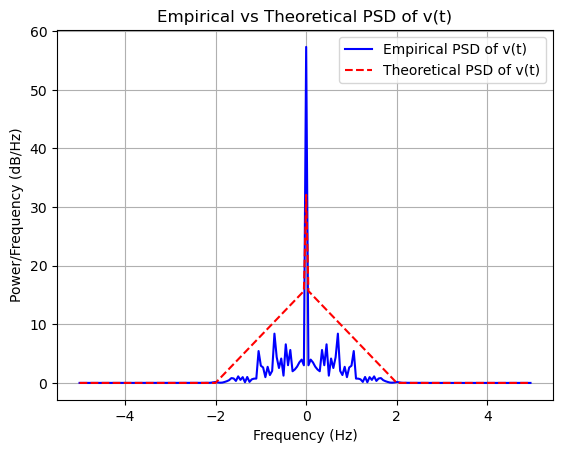
Schonhoff’s Simulation#
import numpy as np
import matplotlib.pyplot as plt
def cnv(b, n):
f0 = b / 2 # Center frequency
ts = 0.1 # Sampling interval
ts2 = ts / 2
fi = 1 / ts # Nyquist frequency
df = 1 / (n * ts) # Frequency resolution
n2 = n // 2
ni2 = n2 * ts
# Time vector centered around zero
t = np.arange(-ni2, ni2, ts)
# Autocorrelation function
r = 4 * np.sinc(2 * t)
# Fourier Transform of the autocorrelation function
h = ts * np.fft.fftshift(np.fft.fft(r, n))
mh = np.abs(h)
# Frequency vector for plotting Sx(f)
f = np.arange(-n2, n2) * df
# Power spectral density Sx(f)
plt.figure()
plt.plot(f, mh)
plt.xlabel('Frequency (Hz)')
plt.ylabel('S_x(f)')
plt.grid(True)
plt.show()
# Convolution to obtain 2C(f)
fc = 2 * np.convolve(h, h) / (n * ts)
# Frequency vector for plotting 2C(f)
fl = np.arange(-len(fc)//2, len(fc)//2) * df
# Power spectral density 2C(f)
plt.figure()
plt.plot(fl, np.abs(fc))
plt.xlabel('Frequency (Hz)')
plt.ylabel('2C(f)')
plt.grid(True)
plt.show()
# Inverse Fourier Transform to obtain autocorrelation of squared process
g = np.fft.ifftshift(np.fft.ifft(fc))
# Time vector for plotting 2Rx(tau)^2
tt = np.arange(-n + 1, n) * ts2
# Autocorrelation Rx(tau)
plt.figure()
plt.plot(t, r)
plt.xlabel(r'$\tau$ (s)')
plt.ylabel(r'$R_x(\tau)$')
plt.grid(True)
plt.show()
# Autocorrelation 2Rx(tau)^2
plt.figure()
plt.plot(tt, np.abs(g))
plt.xlabel(r'$\tau$ (s)')
plt.ylabel(r'$2R_x^2(\tau)$')
plt.grid(True)
plt.show()
return fc
# Example usage:
b = 100 # Bandwidth in Hz
n = 1024 # Number of samples
fc = cnv(b, n)
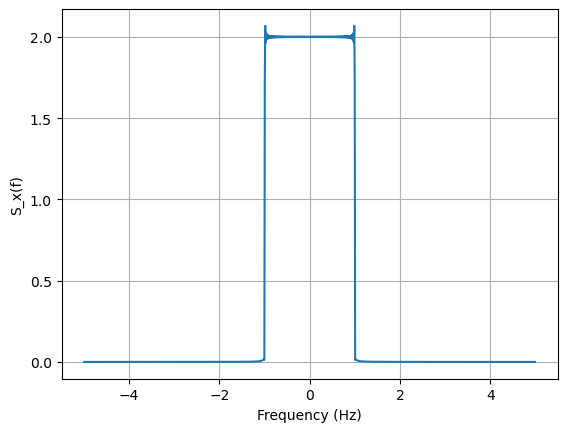
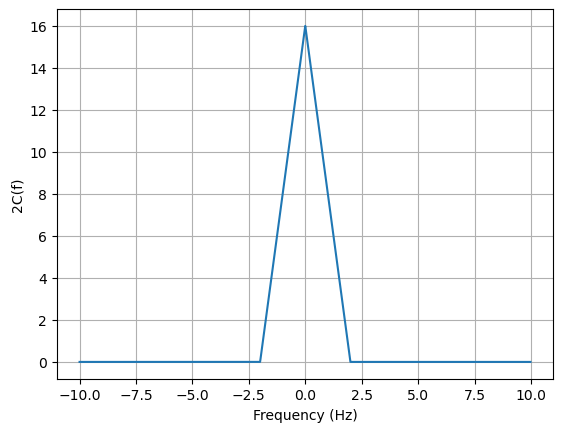
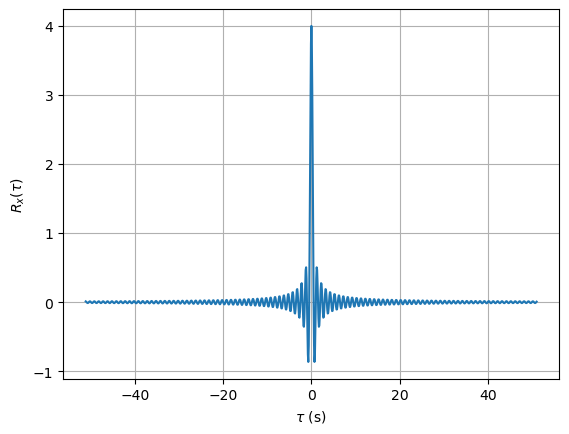
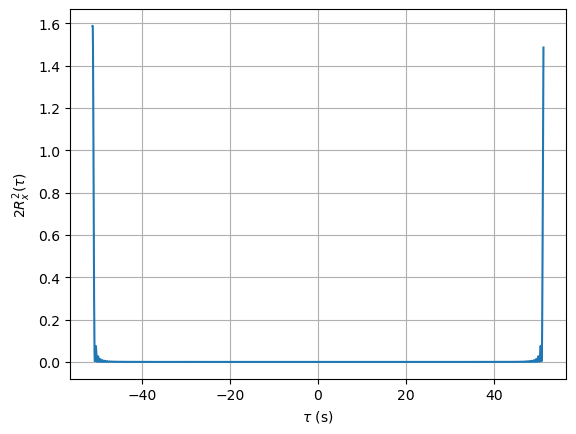